In the world of technology, deepfakes stand out as proof of machine learning’s incredible capabilities – and potential dangers –. As ML engineers and AI researchers we’ve begun a journey to demystify deepfakes, not to alarm you but to arm you with knowledge. Through a series of examples we’ve prepared, we’ll explore the mechanics, implications, and countermeasures surrounding these digital doppelgängers.
The Mechanics of Deepfakes
At their core, deepfakes are hyper-realistic digital imitations created using sophisticated machine learning models, such as Generative Adversarial Networks (GANs). This concept involves two neural networks contesting with each other, where one agent’s gain is another agent’s loss, with one part generating fake images or videos and the other evaluating their authenticity. The main principle of a GAN is based on the indirect training through another neural network that can tell how realistic the input seems, which itself is also being updated dynamically, called the Discriminator. This means that the Generator is not trained to minimize the distance to a specific image but to fool the discriminator instead, allowing the model to learn in an unsupervised way. Through iterative training on vast datasets of real images and videos, these models learn to produce fakes indistinguishable from authentic content to the untrained eye.
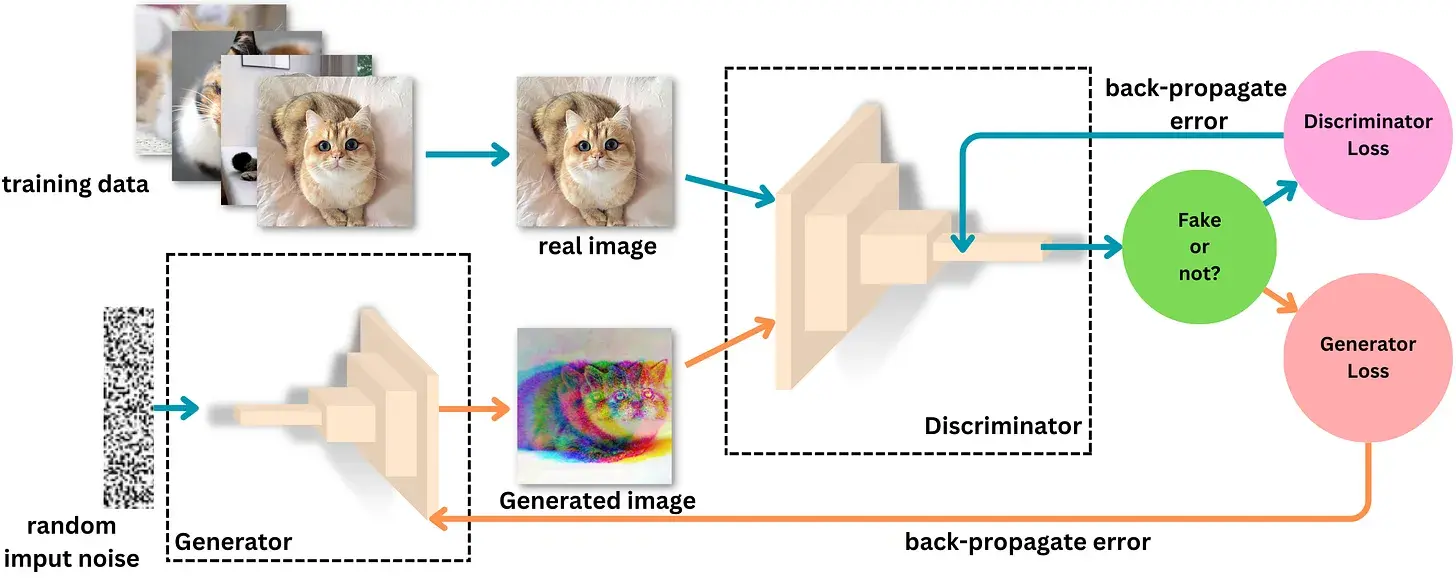
Image showing how Generative Adversarial Networks (GANs) work, with two main parts: the Generator, which creates fake images or videos, and the Discriminator, which tries to tell if they’re real or fake. In this setup, one agent’s success means the other’s failure: if the Generator fools the Discriminator, it wins, but if the Discriminator spots the fake, it comes out on top. This back-and-forth drives both to improve continuously and make the fakes look very realistic.
In addition to these advanced techniques, even simpler methods can create a convincing deepfake in just minutes without having to train a model ourselves. Some tools allow for the cloning of a person’s voice from a short audio sample. This cloned voice can then be synced to a video of choice using technologies such as Wav2Lip, which adjusts the lip movements in the video to match the new audio in a very convincing way. These developments mean that creating deepfakes no longer requires extensive technical expertise or resources, significantly lowering the barrier to entry for those wishing to create digital deception for personal or malicious purposes. This accessibility increases the need for awareness and understanding of deepfakes among the general public, and the development of robust detection and prevention strategies.
Exploring Deepfake Examples
To bring this concept to life, we’ve created a deepfake example designed to show the technology’s capabilities and its potential for misuse. Listen to our CEO, Iago Rodríguez, announcing that we will be receiving a $10 million bonus. (Initially, everyone was thrilled). It took less than an hour to clone his voice from an audio and sync his lips to it in a video. While entirely fictional, the realism of the video serves as a reminder of the power of deepfakes to sow misinformation and chaos.
The Threat to Personal Lives and Society
The dangers posed by deepfakes extend to our personal lives and the broader society we live in. Imagine a deepfake video maliciously crafted to impersonate a loved one, confessing to an act they never committed, or a public figure saying things that upset a lot of people and cause trouble in the community. These scenarios, while fictional, highlight the profound impact deepfakes can have on personal relationships, mental health, and social harmony. Beyond individual distress, deepfakes can manipulate public opinion, incite conflict, and undermine trust in the media and public institutions. The effects can lead to widespread misinformation, polarized communities, and even affect the outcomes of democratic processes. In this light, the need for vigilance and countermeasures against deepfakes becomes not just a technical challenge but a societal imperative to preserve our collective sense of reality and trust.
Detection Techniques and Machine Learning Countermeasures
In response to the deepfake threat, the machine learning community has rallied to develop detection techniques to distinguish real from fake. These methods often focus on subtle inconsistencies in deepfake videos, such as irregular blinking patterns or unnatural speech rhythms. However, as deepfake technology advances, detection becomes a moving target, requiring ongoing research and innovation.
Ethical Machine Learning and the Future of Deepfakes
The battle against deepfakes is not just technical but ethical. We must navigate the fine line between innovation and integrity, ensuring our creations serve the greater good. Looking forward, the future of deepfakes will be shaped by our commitment to ethical AI, with an emphasis on transparency, accountability, and the protection of digital trust. That’s exactly why we’ve put together this blog post and are collaborating with clients to both inform about and prevent the malicious use of deepfakes and how to detect them. We invite you to engage with our efforts, whether by participating in community discussions, sharing this blog post or contributing to open-source detection projects.
How to detect a Deep Fake
There are several challenges and fine details when creating a deepfake. It’s important to know about them to protect us against possible attacks and to protect our data.
Challenges
- Cloning a voice is possible with paid services and open source models, but making it sound natural is a challenge and requires good quality audios. Voice cloning models perform better in English than other languages.
- Not every message fits every video: video generation and voice cloning are two separate steps. It’s crucial to pay attention to the alignment between the message and the image, between the sound and what is said. This is where lip reading becomes vital to the process.
- The eyes and mouth are the most challenging areas to make look realistic and synchronized with the audio.
- There are numerous services available for each step (see the slide for a step-by-step guide).
Key Findings
- Importance of data: high-quality audio and video of the person are essential for producing a convincing deepfake. Be sure to protect your data if you are not a public exposed person.
- Error detection: identifying and correcting issues like desynchronization and visual artifacts is a meticulous process. Pay attention to small details to detect well executed deepfakes.
- Achieving realism: the final deepfake achieves a high level of realism through the precise integration of filler words and gesture synchronization. Starting with a video makes it easier to create a speech that matches the gestures.
- Top tools: ElevenLabs excels at realistic voice cloning, and SyncLabs is the best for syncing the generated audio with the video.
Step-by-Step Guide to Create a Deepfake:
- Choose a high-quality base video.
- Create a script for the audio that aligns with the video’s gestures, including filler words to enhance realism.
- Select a clean audio recording of the person speaking, free from background noise, with a minimum length of one minute. Clone the voice using services like Eleven Labs.
- Apply video edition techniques to synchronize audio and video, and reduce artifacts.
- Generate the deepfake by combining the audio and video using Sync Labs.
- (Optional) Train an existing model with additional videos of the person to improve accuracy and realism.
- (Optional) Manually adjust with video editing tools.
Conclusion
Deepfakes pose a significant threat, blurring the line between reality and deception with potentially severe consequences. However, we can safeguard our digital world with the right knowledge and tools. To protect against misuse, it is crucial to secure images, videos, and audio data and to redact or anonymize personally identifiable information (PII) to minimize risks in the event of a data breach. Always double-check the source of any information and avoid trusting non-official channels, which are often exploited to spread false or misleading content. By staying informed, vigilant, and actively engaged, we can protect our digital identities and ensure the future of machine learning remains secure and trustworthy.
Explore further the world of deepfakes with us. Visit leniolabs.com for resources, tutorials, and more on how you can get involved.
—
Follow us on social media (/@leniolabs_)
🐦 x.com/leniolabs_
🔗 linkedin.com/company/leniolabs
▶️ youtube.com/leniolabs
Or sign up for our Last Week on AI newsletter to stay at the front line of machine learning and AI news.
🗞️ https://medium.com/leniolabs
Let’s shape a future where technology empowers, not endangers, our digital lives.